Policy Evaluation Analysis For Roles of State in Asymmetric Partially Observable RL
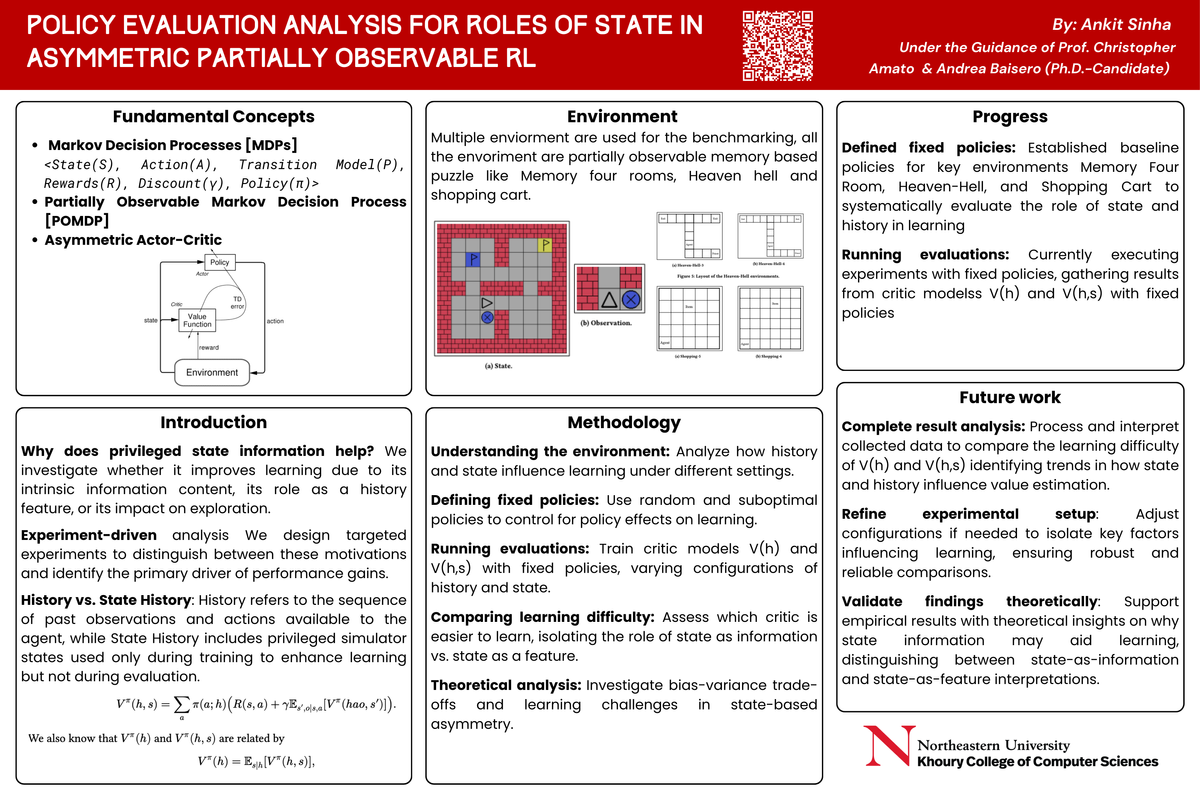
Introduction:
In reinforcement learning, agents typically rely on their history—past observations and actions—to make decisions. However, some training frameworks leverage privileged state information, available only during training, to enhance learning. But why does this additional information help?
Our research explores three key hypotheses:
- State as Information – A Privileged state provides valuable knowledge beyond history, improving learning efficiency.
- State as a Feature – The state serves as a structured representation of history, making learning easier.
- State for Exploration – Access to state information during training enhances exploration, leading to better policy learning.
To test these ideas, we design experiment-driven analyses, comparing critic models trained with history alone versus those using both history and privileged state. By examining learning difficulty, performance differences, and bias-variance trade-offs, we aim to pinpoint the primary driver of performance gains.
Through this study, we hope to deepen our understanding of state-based asymmetry and its role in reinforcement learning, paving the way for more effective offline training strategies. Stay tuned for our findings!
References:
- Andera's Asymmetric Actor Critic Paper: arXiv:2105.11674
- Github Repo (Main):https://github.com/abaisero/asym-rlpo
- Mine Github Repo: https://github.com/Ank-22/asym-rlpo/tree/feature/evaluation-algorithms